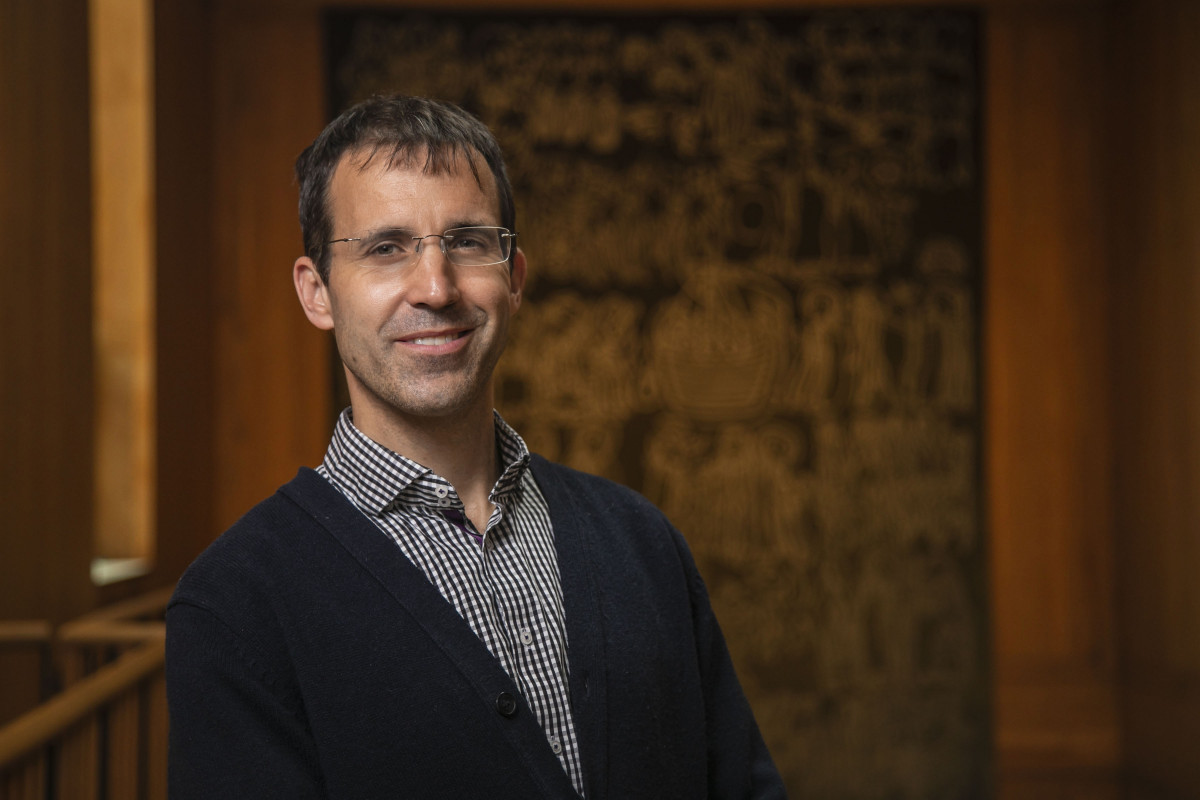
Cal Muckley
University College Dublin
Resume
Cal is Professor of Operational Risk in Banking and Finance at the UCD College of Business and a Fellow at the UCD Geary Institute. His research is in areas of financial misconduct, mitigating financial fraud and the information content of corporate dividends. He has published in international scholarly journals including the Journal of Corporate Finance, the Journal of Econometrics and the Journal of Empirical Finance. Previously he has worked as a lecturer in finance at the Department of Economics and Finance, Durham Business School. He obtained his Ph.D. degree from Trinity College Dublin. He has been a Visiting Scholar at New York University Stern School of Business, Yale University, at Groningen and Tilburg Universities in the Netherlands, at ESC Rennes School of Business in France, at Freiburg University and at the London School of Economics. He is a Fulbright Scholar and he has also received other awards and fellowships. These include a Thomas Moran Fellowship from the Mutual of America Life Insurance, a Research Impact Award from UCD and the Barrington Medal from the Statistical & Social Inquiry Society. His work has been widely reported in the financial press, including in the Wall Street Journal.
Abstract
Countering racial discrimination in algorithmic lending: A case for model-agnostic interpretation methods
C Muckley with A Aggarwal and P Neelakantan
In respect to racial discrimination in lending, we introduce global Shapley value and Shapley-Lorenz explainable AI methods to attain algorithmic justice. Using 157,269 loan applications during 2017 in New York, we confirm that these methods, consistent with the parameters of a logistic regression model, reveal prima facie evidence of racial discrimination. We show, critically, that these explainable AI methods can enable a financial institution to select an opaque creditworthiness model which blends out-of-sample performance with ethical considerations. Policy implications of the potential blending of out-of-sample performance and ethical considerations are highlighted.