8th European COST Conference on Artificial Intelligence in Finance


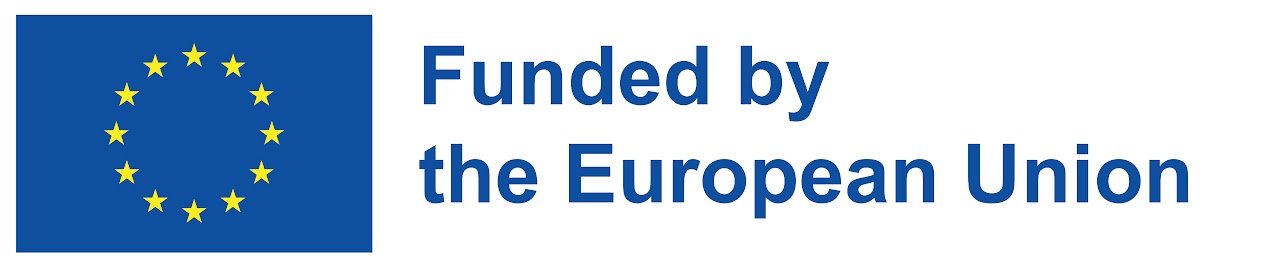

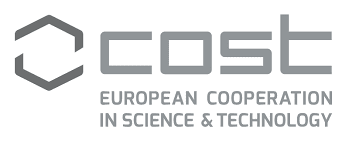

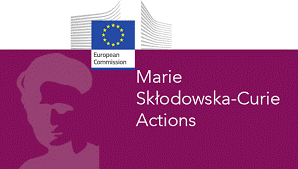
Humane Digital Transformation
- Main Research Conference: Sept 29, 9am to 5pm
- Annual Management Committee Meeting COST FinAI: Sept 28, 9am to 5pm
- Annual Working Group Meetings COST FinAI: Sept 27, 9am to 5p
Joint with the Marie Sklodowska-Curie Action Doctoral Network on Digital Finance
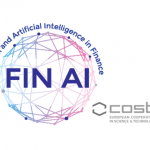
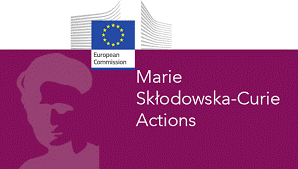
Summary
Forum for interdisciplinary discussions and exchange of ideas on the adoption of innovative technologies in finance, bringing together academic and industry experts. The topics we will cover include (but are not limited to):
- Opportunities for AI adoption by the financial sector;
- Challenges emerging from the fast adoption of novel technologies in the provision of financial services;
- State of the art solutions.
- The conference is for free. We would kindly ask you to register: Conference Registration
The COST Action Fintech and Artificial Intelligence in Finance
The COST (Cooperation in Science and Technology) Action 19130
51 countries, 200+ researchers from 100+ universities
Funded by the Horizon Europe Framework Programme of the EU
The network
Management Committee, Working group members
The Research Topics
Fintech, Artificial Intelligence in Finance, Transparency in Finance, Machine Learning, Blockchain, Cryptocurrencies
How to join our COST Action
Sign up for working group membership here.
Conference Chairs and Organization Team
Prof. Dr. Branka Hadji Misheva, Professor of Applied Data Science and Finance, BFH, Switzerland
Prof. Dr. Jörg Osterrieder, Professor of Sustainable Finance, BFH, Switzerland and Associate Professor of Finance and Artificial Intelligence, University of Twente, Netherlands
Prof. Dr. Christian Hopp, Professor of Finance, BFH, Switzerland , Head of Institute Applied Data Science and Finance
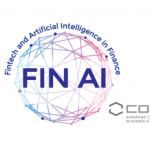
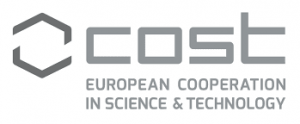
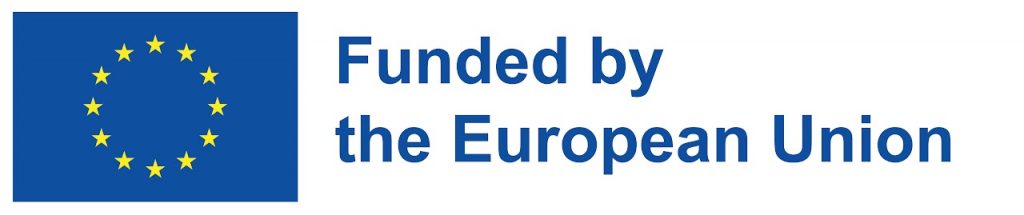
Schedule
September 29, 2023 | |
European COST Conference on AI in Finance | September 29 2023, 08:30 – 17:30, (BFH Bern Business School and online) |
08:30 – 09:30 | Registration and Check-In |
09.30 – 09.45 | Welcome and Opening |
Prof. Dr. Christian Hopp, Prof. Dr. Branka Hadji Misheva, and Prof. Dr. Jörg Osterrieder | |
Session Finance I | Chair: Prof. Dr. Branka Hadji Misheva |
09:45 – 10:15 | Quantinar: A Blockchain p2p Ecosystem for Scientific Research I Prof. Dr. Wolfgang Härdle (Humboldt University Berlin) |
10:15 – 10:45 | Next-gen quant investing I Harald Lohre (Robeco) |
10.45 – 11.00 | Coffee break |
11.00 – 11.30 | Graph Machine Learning for Financial Crime Analysis I Kubilay Atasu (IBM Research) |
11.30 – 12.00 | Use of regimes to tackle uncertainty in capital market assumptions I Jakub Rojček (LGT Private Banking Europe) |
12.00 – 13.00 | Lunch break |
Session Finance II | Chair: Prof. Dr. Christian Hopp |
13.00 – 13.30 | Regulatory Change Management – a practical example of support by machine learning capabilities I Philipp Hartmann (Credit Suisse) |
13.30 – 14.00 | Responsible Machine Learning in Finance: Data Quality, Model Transparency and Responsibility, and the EU AI Act I Nicole Königstein (Wyden Capital AG) |
14.00 – 14.30 | Using Machine Learning for predicting Consumer Loan Default: A case study for US Digital Lenders I Arben Zibri (Cardo AI) |
14.30 – 15.00 | Prof. Dr. Ali Hirsa (Columbia University) |
15.00 – 15.30 | Coffee break |
Session Finance III | Chair: Prof. Dr. Jörg Osterrieder |
15:30 – 15:50 | Network-based scoring models for P2P Systems I Prof. Dr. Branka Hadji Misheva |
15:50 – 16:10 | Share Buybacks – The Multi-billion dollar question I Prof. Dr. Jörg Osterrieder |
16:10 – 16:30 | Closing remarks: Artificial Intelligence in Finance – The European COST Network |
16:30 – 17:30 | Apero |
September 28, 2023 | |
Management Committee meeting | September 28 2023, 8:30 – 15:00, (BFH Bern Business School and online) |
Chair: Prof. Dr. Jörg Osterrieder | |
8:30 – 9:30 | Registration and Check-In |
9:30 – 10:15 | Opening and introduction of the agenda |
Prof. Dr. Jörg Osterrieder, Prof. Dr. Branka Hadji Misheva | |
10:15 – 12:00 | Updates from Working Groups, Grant Holder, STSM Coordinators, Diversity Team, Sciene Communication Coordinator |
12:00 – 13:30 | Lunch |
13:30 – 16:30 | Country Updates |
16:30 | Walk/break |
18:00 – 20:00 | Speakers’ dinner |
September 27, 2023 | |
Working and core group meetings | September 27 2023, 8:30 – 16:00, (BFH Bern Business School and online) |
Session COST FinAI | Chair: Prof. Dr. Jörg Osterrieder, Prof. Dr. Branka Hadji Misheva |
8:30 – 9:30 | Registration and Check-In |
9:30 – 10:00 | Core group meeting |
Prof. Dr. Jörg Osterrieder, Prof. Dr. Branka Hadji Misheva | |
10:00 – 10:30 | Working Group 3 Meeting and Presentations |
Prof. Dr. Peter Schwendner | |
11:00 – 11:30 | Working Group 2 Meeting and Presentations |
Prof. Dr. Petre Lameski | |
11:30 – 12:00 | Lunch & Working Group 1 Meeting and Presentations |
Prof. Dr. Wolfgang Karl Härdle | |
12:00- 18:00 | Country Updates & Excursion to Lauterbrunnen |
September 26, 2023 | |
11:00 – 18:00 | Working Excursion to the mountains |
Programme Committee
Prof. Dr. Christian Hopp, BFH Bern University of Applied Sciences, Switzerland
Prof. Dr. Branka Hadji Misheva, Zurich University of Applied Sciences, Switzerland
Prof. Dr. Jörg Osterrieder, BFH Bern University of Applied Sciences, Switzerland and University of Twente, Netherlands
Bios and Abstracts
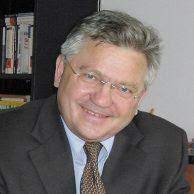
Prof. Dr. Wolfgang Karl HÄRDLE attained his Dr. rer. nat. in Mathematics at Universität Heidelberg in 1982 and in 1988 his habilitation at Universität Bonn. He is Ladislaus von Bortkiewicz Professor of Statistics at Humboldt-Universität zu Berlin and the director of the Sino German Graduate School (洪堡大学 + 厦门大学) IRTG1792 on “High dimensional non stationary time series analysis”. He also serves as head of the joint BRC Blockchain Research Center (with U Zürich & U Bukarest). He is guest professor at SMU, ACI NUS, Singapore, NYCU, Hsinchu TW, Charles U, Prague CZ.
His research focuses on data sciences, dimension reduction and quantitative finance. He has published over 30 books and more than 300 papers in top statistical, econometrics and finance journals. He is highly ranked and cited on Google Scholar, REPEC and SSRN. He has professional experience in financial engineering, SMART (Specific, Measurable, Achievable, Relevant, Timely) data analytics, machine learning and cryptocurrency markets. He has created a financial risk meter, FRM hu.berlin/frm, a cryptocurrency index, CRIX royalton-crix.com. He invented the Q2 eco system quantlet.com & quantinar.com Together with the BRC blockchain-research-center.com he has created IDA Institute for Digital Assets, ASE, RO. His web page is:hu.berlin/wkh
Quantinar: A Blockchain p2p Ecosystem for Scientific Research
Abstract. Living in the Information Age, the power of data and correct statistical analysis has never been more prevalent. Academics and practitioners require nowadays an accurate application of quantitative methods. Yet many branches are subject to a crisis of integrity, which is shown in an improper use of statistical models, $p$-hacking, HARKing, or failure to replicate results. We propose the use of a Peer-to-Peer (P2P) ecosystem based on a blockchain network, Quantinar (quantinar.com), to support quantitative analytics knowledge paired with code in the form of Quantlets (quantlet.com) or software snippets. The integration of blockchain technology makes Quantinar a decentralized autonomous organization (DAO) that ensures fully transparent and reproducible scientific research.
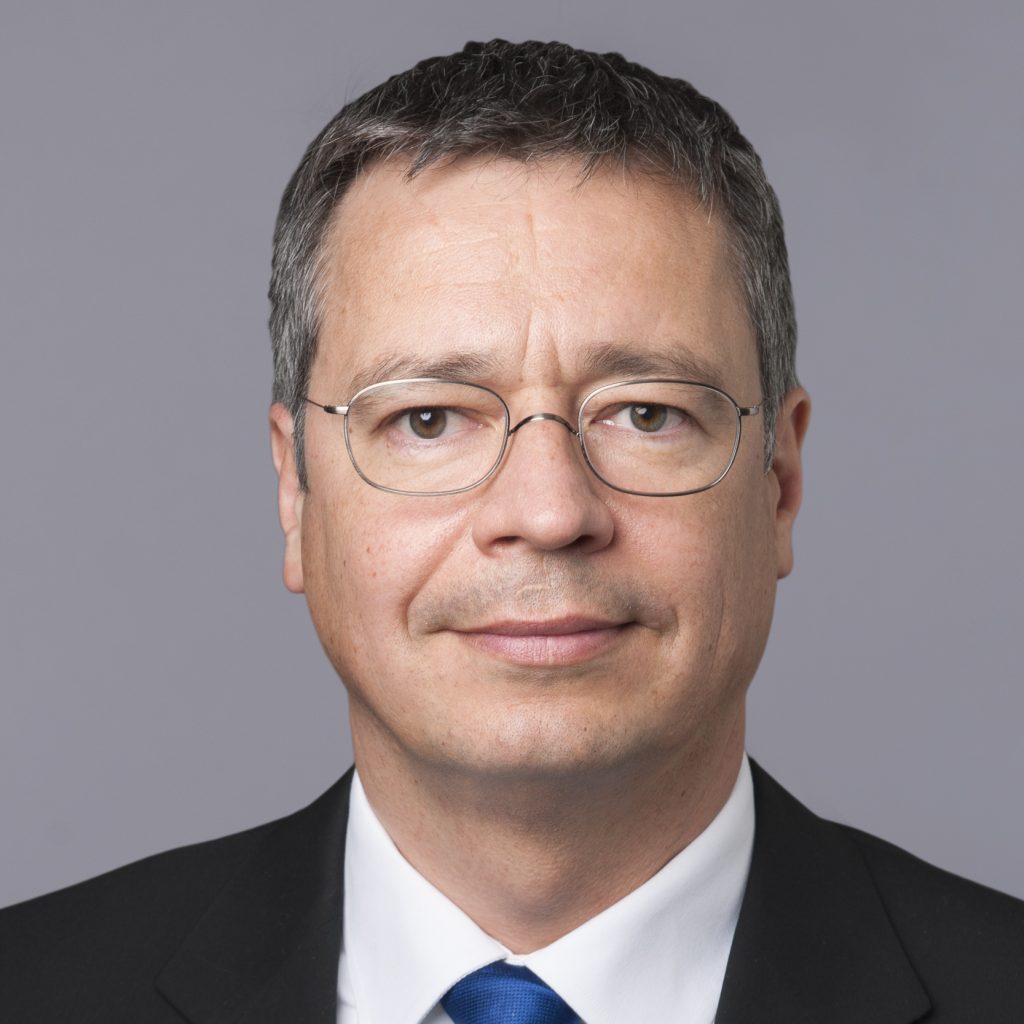
Philipp Hartmann is a Managing Director of Credit Suisse in the Public Policy & Regulatory Foresight function, based in Zürich, Switzerland. He is leading the global Regulatory Foresight and Intelligence function. He joined Credit Suisse in January 2006 and held various roles in the areas of Regulatory Change Management, Business Development, Investment Products as well as Advisory Process. Prior to joining Credit Suisse, he was Head of Financial Services Practice and Partner, at Arthur D. Little in Switzerland.
Philipp holds a Ph. D. in economics and a master degree in Business Administration and Mechanical Engineering from the Technical University of Darmstadt, Germany.
Regulatory Change Management – a practical example of support by machine learning capabilities
Abstract: This practical example illustrates the application of machine learning capabilities along the regulatory change management process. Starting with an overview of the end-to-end processes, the presentation focusses on the use of machine learning to support analysts in the assessment regarding applicability of upcoming regulations. As the volume of regulatory events relevant for a global bank is large, so machine learning can assist the analysts. The applied machine learning model provides for a likelihood of the relevance of the regulatory event for a given pre-defined category as a support.
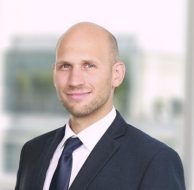
Dr. Jakub Rojcek (Slovak/Swiss) is a Head of Quantitative Analysis of LGT Private Banking EMEA based in Zurich. He joined LGT Group in asset management unit called Capital Partners in 2016 in Pfäffikon. He is reponsible for Strategic asset allocation and Capital market assumptions at LGT Private Banking EMEA alongside other quantitative topics like Quantitative tactical cross-asset strategy.
He completed his Ph.D. in Finance at the University of Zurich and Swiss Finance Institute and holds Master’s and Bachelor’s degrees from the University of Bonn and Charles University in Prague, respectively. He is fluent in Slovak, English, German and Czech
Use of regimes to tackle uncertainty in capital market assumptions
Abstract: Optimization of long-term portfolios requires estimates of expected returns and risk for many asset classes. These are collectively known as capital market assumptions. Such estimates are inherently uncertain and portfolio optimization requires use of robust techniques. We show how regime indicators can be used to create uncertainty sets which can then be used in portfolio optimization. In the talk, we also sketch, how the recent developments in NLP and AI can be incorporated within regime indicators.
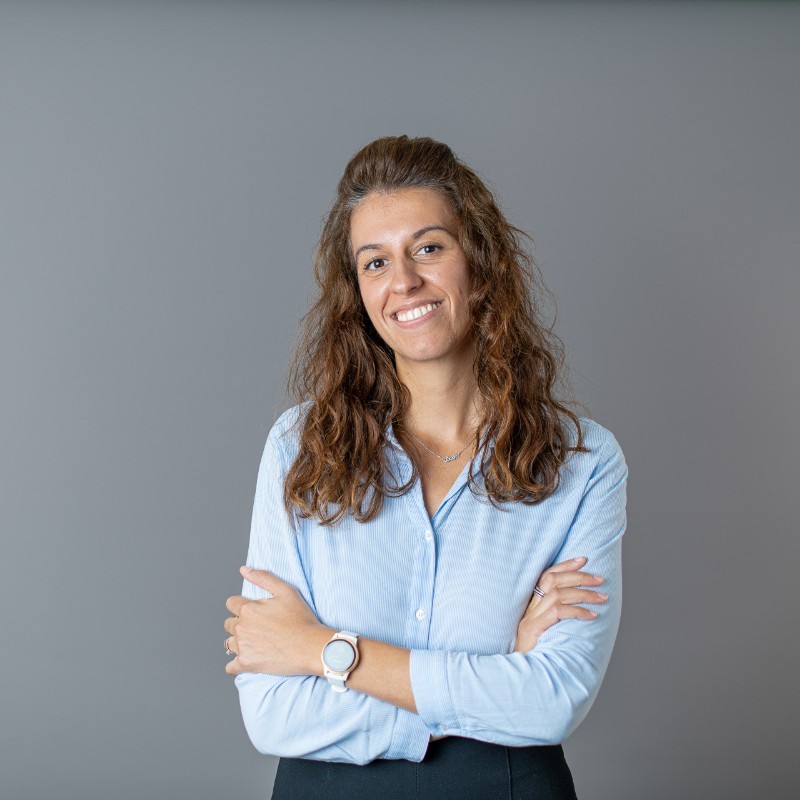
Prof. Dr. Branka Hadji Misheva (Macedonian) is a Professor in Applied Data Science & Finance. Her primary responsibilities include teaching data analytics, digital finance, AI, and ML for finance. She also plays a pivotal role as a Scientific Grant Holder for the COST Action, focusing on “FinTech and Artificial Intelligence in Finance: Towards a transparent financial industry”. Additionally, she has embarked on research and innovation projects centered on ML-based scoring models and eXplainable AI for finance.
Her research specializations encompass Machine Learning-based scoring models for P2P systems, eXplainable AI for finance, application of network theory in fintech risk management, and addressing biases in ML models for credit risk management.
Prof. Dr. Hadji Misheva’s professional journey began as a Data Scientist in banks and IT companies. She then transitioned to the role of Senior Researcher and Technical Project Manager of the Horizon 2020 Project at the University of Pavia. Her most recent position was as a Senior Researcher/Scientific Employee at the Zurich University of Applied Sciences.
In the realm of academics, she achieved her PhD in Economic and Management of Technology from the University of Pavia, Italy. She also holds an MBA in Global Business and Sustainability from Universita Cattolica, Italy, an MSc in Economics for Business Analysis from Staffordshire University, United Kingdom, and a BSc in Financial Management from the Faculty of Economics, Skopje, Macedonia.
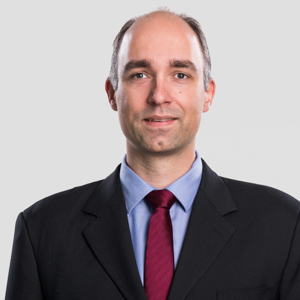
Prof. Dr, Joerg Osterrieder is Professor of Sustainable Finance at Bern Business School in Switzerland and Associate Professor of Finance and Artificial Intelligence at the University of Twente in the Netherlands. He has more than 15 years of experience in financial statistics, quantitative finance, algorithmic trading, and the digitization of the finance industry. Joerg is the Chair of the European COST Action 19130 Fintech and Artificial Intelligence in Finance, an interdisciplinary research network comprised of over 300 researchers from 51 countries globally. As the Coordinator for the nominated Marie Sklodowska-Curie Action Industrial Doctoral Network on Digital Finance, Joerg chairs a consortium comprised of 18 distinguished partners from both academic and industrial sectors throughout Europe, dedicated to enhancing and fortifying research and PhD-level education. He is a founding associate editor of Digital Finance, editor of Frontiers Artificial Intelligence in Finance, and frequent reviewer for leading academic journals. He also serves as an expert reviewer for the European Commission’s “Executive Agency for Small and Medium-Sized Enterprises” and “European Innovation Council Accelerator Pilot” programs. He was the director of studies for an executive education course titled “Blockchain, Mahine Learning, and Data Science in Finance” and the primary organizer of a series of annual research conferences on Artificial Intelligence in Finance. In close collaboration with the Finance industry, he has led or co-led over thirty national and international research projects on a wide range of quantitative, data-driven topics over the past few years. Previously he worked as an executive director at Goldman Sachs and Merrill Lynch, as quantitative analyst at AHL as well as a member of the senior management at Credit Suisse Group. Joerg is now active at the intersection of academia and industry, focusing on the implementation of research results in the financial services industry.
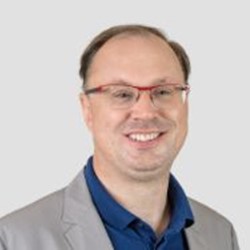
Prof. Dr. Christian Hopp (German) is currently the head of the Institute for Applied Data Science and Finance at BFH. His professional focus revolves around Entrepreneurship, Innovation Management, Entrepreneurial Financing, Econometrics, as well as Big Data Analysis and Artificial Intelligence.
Christian’s educational journey spans across several countries. He embarked on his study of business administration in Germany, the Netherlands, and the USA. He accomplished his doctorate in quantitative economics and finance from the University of Konstanz. Further refining his expertise, he pursued his master’s degree in business administration from two esteemed institutions: the University of Pittsburgh (MBA) and the Rotterdam School of Management (Master in Financial Management).
Christian has an impressive academic trajectory. Before his current role at BFH, which began in July 2020, he headed the teaching and research area of Technology-oriented Entrepreneurship at RWTH Aachen University. Prior to this, from 2008 to 2013, he held the position of assistant professor at the University of Vienna. His primary research interests are rooted in start-up management, start-up financing, and personnel economics.
For those keen on exploring more about his academic contributions, he maintains active profiles on academic platforms. You can delve deeper into his work on [Google Scholar](https://scholar.google.de/citations?user=GAz_E1IAAAAJ&hl=en&oi=sra) and [ResearchGate](https://www.researchgate.net/profile/Christian_Hopp).
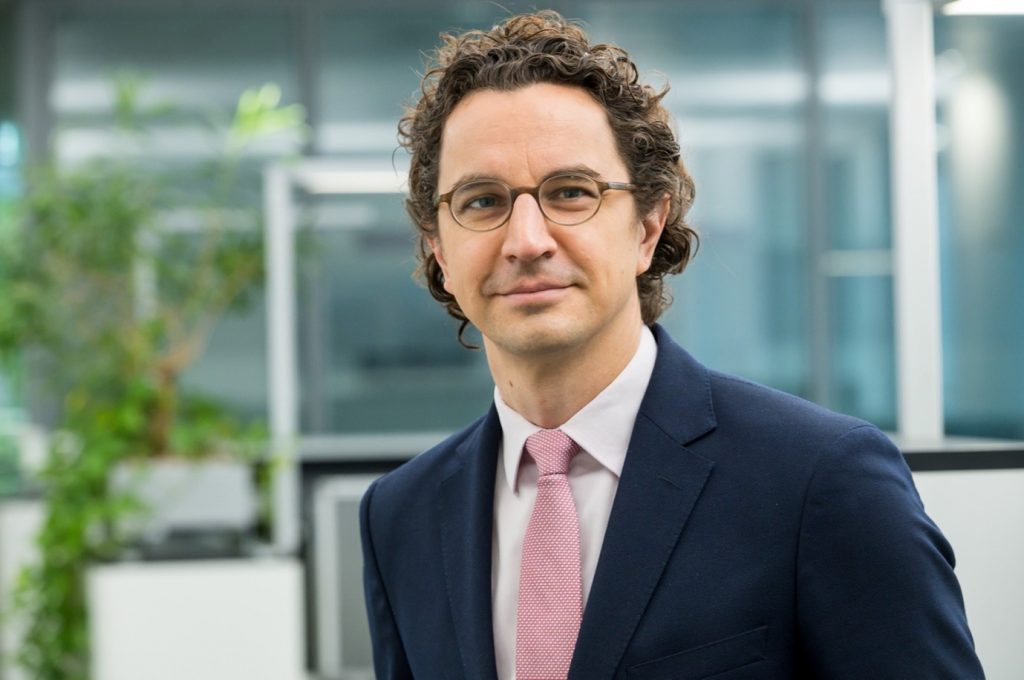
Dr. Harald Lohre is Executive Director of Research in the Quant Equity Research Management Team of Robeco. He is also an Honorary Researcher at Lancaster University Management School as well as a Research Fellow at the Hamburg Financial Research Center and he has published in the Journal of Empirical Finance, the Financial Analysts Journal, and the Journal of Portfolio Management. Prior to joining Robeco in 2022, Harald served on the management team of Invesco Quantitative Strategies and was Head of Quantitative Research and Portfolio Manager at Deka Investment. Harald holds a Doctorate in Finance summa cum laude from the University of Zurich and a Diploma in Mathematical Finance from the University of Konstanz. He is a past Fellow of the Centre for Endowment Asset Management at Cambridge Judge Business School and serves on the Research Committee of Inquire Europe and as Associate Editor of the Journal of Systematic Investing.
Next-gen quant investing
Abstract: Quantitative investing has always been leveraging quantitative tools to translate data into informed investment decisions. In this talk, we explore how quantitative investing is evolving given the exciting advances in data, algorithms, and computing power. We discuss the promises and pitfalls of machine learning and NLP, and how these techniques can help advance incumbent factor investing processes as well as conceive next gen quant investing designs. The underlying objective is not just to optimally trade off financial risk and return, but to effectively integrate and support sustainability within the investment process.
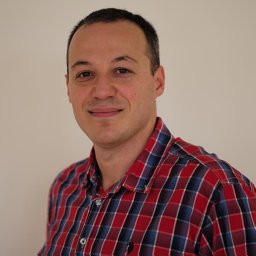
Dr. Kubilay Atasu is a senior member of IEEE and a senior research scientist at IBM Research – Zurich. He joined IBM in 2008, where he has been developing high-speed text analytics, graph analytics, and machine learning solutions. While with IBM, he taught post-graduate courses at the University of Tübingen and at the Sabanci University, Istanbul. In addition, he served in the program committees of more than 50 scientific conferences and co-chaired two IEEE conferences. He is the principal investigator of a Swiss National Science Foundation (SNF) project aimed at building scalable graph ML solutions for financial crime analysis.
Graph Machine Learning for Financial Crime Analysis
Abstract: Graph machine learning (ML) is an emerging field of Artificial Intelligence motivated by the ubiquity of graph-structured data in real-life applications. Graph neural networks (GNNs) serve as a key technology in this area, and can be leveraged in various fields including the financial industry. In this talk, I will cover applications of graph machine learning to financial crime analysis tasks, including anti-money laundering and fraud detection. I will present a scalable graph machine learning library, which enables real-time financial transaction and account activity monitoring on IBM’s z16 processors. Next, I will discuss how GNNs and Large Language Models (LLMs) can help address some key technological problems in the financial crime analysis space and the challenges of deploying such solutions in commercial offerings.
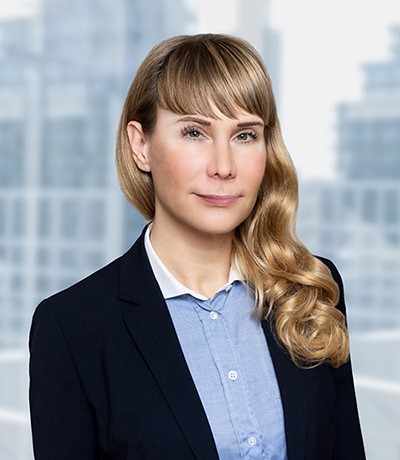
Nicole Königstein is a distinguished Data Scientist and Quantitative Researcher, currently working as Chief Data Scientist and Head of AI & Quantitative Research at Wyden Capital and as Head of AI and Quantitative Research at quantmate. Alongside her role in this organization, she serves as an AI consultant across diverse industries, leading workshops, and guiding companies from the conceptual stages of AI implementation through to final deployment.
As a guest lecturer, Nicole shares her expertise in Python, machine learning, and deep learning at various universities. She is a regular speaker at renowned AI and Data Science conferences, where she conducts workshops and educational sessions. In addition, she is an influential voice in the data science community, regularly reviewing books in her field and offering her insights and critiques. Nicole is also the author of Math for Machine Learning published by Manning Publications and the author of the (forthcoming) book Transformers in Action published by Manning Publications.
Responsible Machine Learning in Finance: Data Quality, Model Transparency and Responsibility, and the EU AI Act
Machine learning and deep learning are significantly influencing the financial industry, making responsible usage of these technologies critical. This talk addresses crucial aspects such as data quality, model transparency and reliability, bias mitigation, content control in LLMs, and the upcoming EU AI Act.
The talk starts by outlining methods for ensuring data quality, emphasizing the importance of data validation for creating reliable and ethical machine learning models. Next, the focus shifts to transparency in machine learning models, extending from traditional models to deep learning and Large Language Models (LLMs). Techniques like local model interpretation and attention visualization will be discussed as ways to understand model behavior better.
The presentation also discusses how to control the content generated by LLMs effectively. Techniques like reinforcement learning from human feedback (RLHF) and token penalization will be explored to help manage the content produced by these models. The talk concludes by addressing ways to identify and reduce bias in machine learning models.
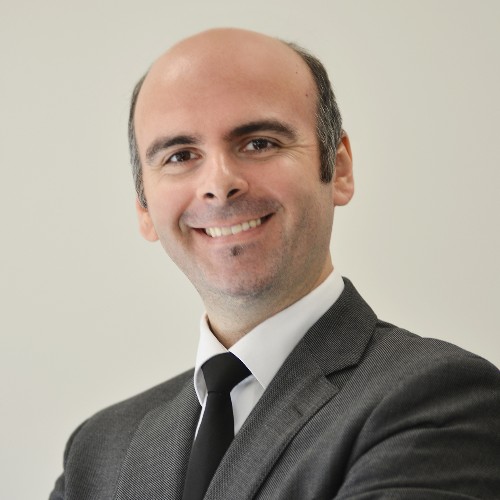
Arben Zibri serves as the Product Manager for the Data Science division at Cardo AI, where he spearheads the articulation of product vision. In this capacity, he is responsible for identifying emerging opportunities and application scenarios that can be addressed through methodologies in Machine Learning and Artificial Intelligence within the realm of structured finance. Cardo AI’s Intelligence business specializes in developing real-time analytical models, leveraging Natural Language Processing, Unstructured Data Modeling, Tabular Data Modeling, and Advanced Quantitative Optimization techniques.
Prior to his affiliation with Cardo AI, Arben occupied the role of Director of Model Control for Interest Rates and Credit Derivatives at Morgan Stanley. Earlier in his professional trajectory, he undertook various roles in project management and commercial lending.
In addition to his professional commitments, Arben serves as an adjunct faculty member, teaching finance-related courses at EPOKA University and the University of New York in Tirana. He is the holder of a Doctorate Degree in Economics from the ‘Aleksander Moisiu’ University in Durres, Albania, and holds the Chartered Financial Analyst (CFA) designation.
Using Machine Learning for predicting Consumer Loan Default: A case study for US Digital Lenders
Abstract: The paper presents the application of tabular data modeling techniques to predict Probability of Default for US Consumer Loans. We use gradient boosting tree models. The study describes the data splitting approach, the model calibration approach, the data available and the performance of the model as compared with the Fico Score. The data used are consumer loan portfolios of digital lenders operating in the US. The case study provides highlights on feature impact to default probability, grouped by characteristics related to the borrower, characteristics related to the loan, and macroeconomic inputs. The paper shows that the model outperforms in terms of PD prediction accuracy Fico Score.
Info and Location
The conference is hosted at the BFH Bern University of Applied Sciences.
The Institute for Applied Data Science & Finance aims to establish itself as a leading Swiss research institute for data-driven, finance-based and strategic insights, analysis and value creation. To this end, an interdisciplinary team of around 25 researchers conducts research and teaching on topics relating to data science, data ethics, data and technology management, data-based business models, corporate financing, digital financing, taxation, accounting and financial reporting.
Bern University of Applied Sciences
Brückenstrasse 73
3005 Bern
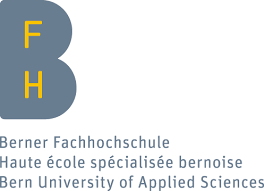
Registration
The conference is for free. We would kindly ask you to register: Conference Registration
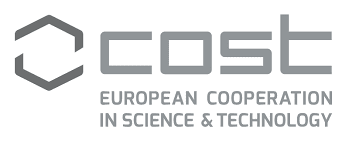
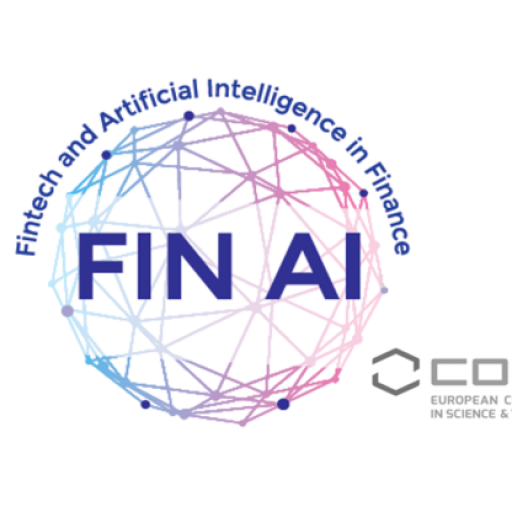
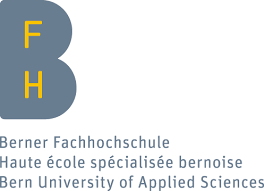
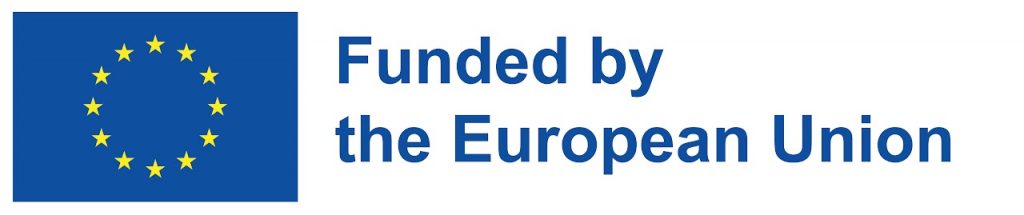
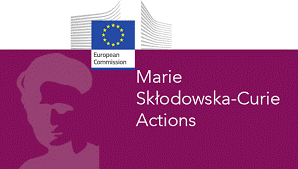
The conference is hosted at the BFH Bern University of Applied Sciences.
The Institute for Applied Data Science & Finance aims to establish itself as a leading Swiss research institute for data-driven, finance-based and strategic insights, analysis and value creation. To this end, an interdisciplinary team of around 25 researchers conducts research and teaching on topics relating to data science, data ethics, data and technology management, data-based business models, corporate financing, digital financing, taxation, accounting and financial reporting.
Bern University of Applied Sciences
Brückenstrasse 73
3005 Bern